Ruijie Zheng
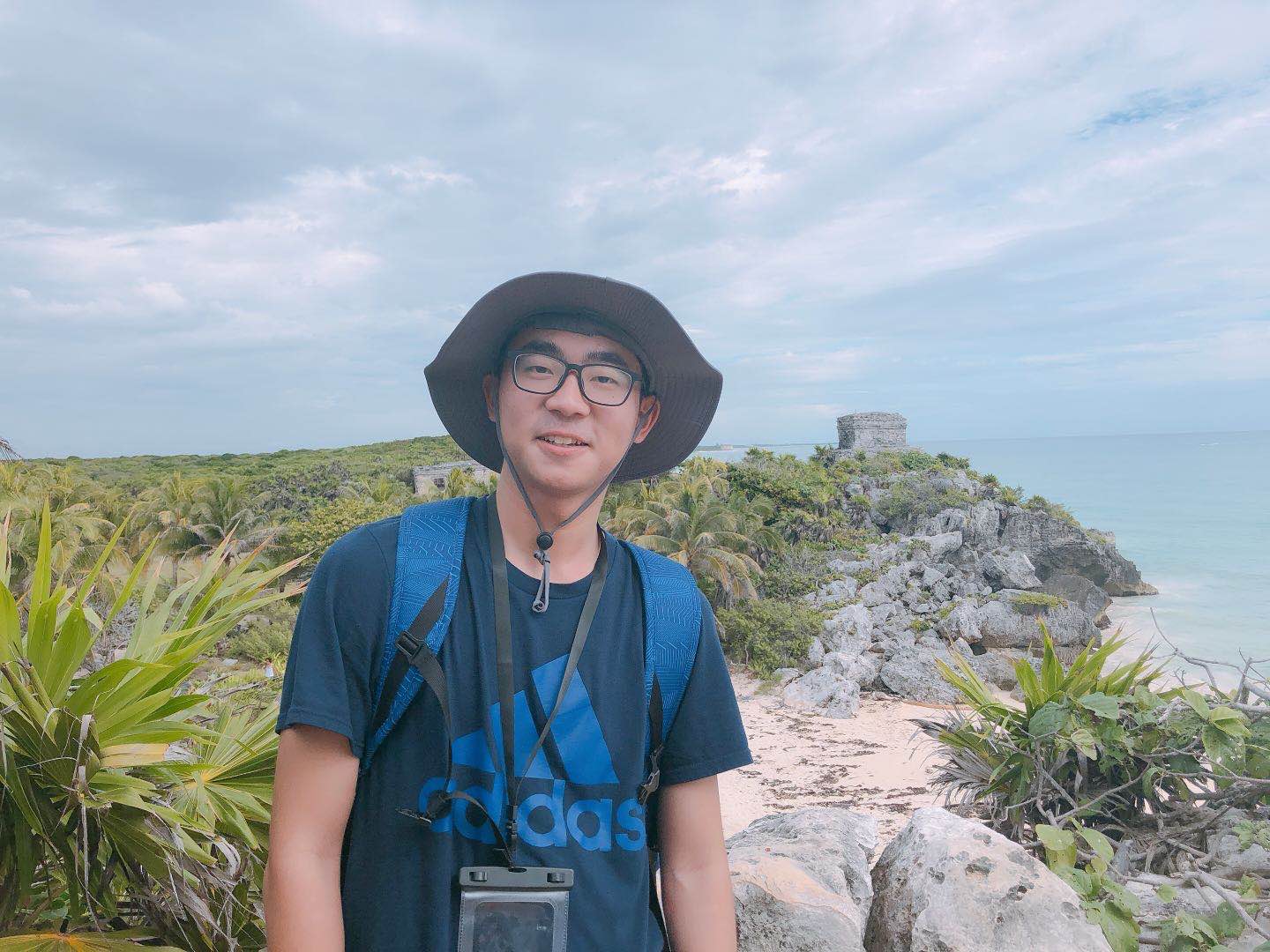
I am a third-year Ph.D. student in Computer Science at the University of Maryland, College Park, where I am fortunate to be advised by Prof. Furong Huang and Prof. Hal Daumé III. Currently, I am a research intern at NVIDIA GEAR lab working with Jim Fan, Yuke Zhu, and Scott Reed on generalist humanoid robot foundation model. Before that, I obtained my Bachelor’s degree in Computer Science and Mathematics both with high honors from the University of Maryland, College Park.
My research spans a variety of topics in reinforcement learning and robotics, including large scale generalist policy pretraining, integrating (latent) world model into robot policy learning, self-supervised representation learning in reinforcement learning and robotics, etc. My long-term goal is to develop a generally capable, robust, and self-adaptive embodied agent, endowed with extensive prior knowledge from a broad spectrum of structured and unstructured data. You can find my CV here.
selected publications
- ICLR 2025TraceVLA: Visual Trace Prompting Enhances Spatial-Temporal Awareness for Generalist Robotic PoliciesIn International Conference on Learning Representations , 2025
- CVPR 2025Magma: A Foundation Model for Multimodal AI Agents2025